Hi, I'm Tobias Sterbak
I'm a freelance data scientist and machine learning engineer focusing on natural language processing (NLP) and LLMs. I like to build smart and useful applications with and without machine learning.
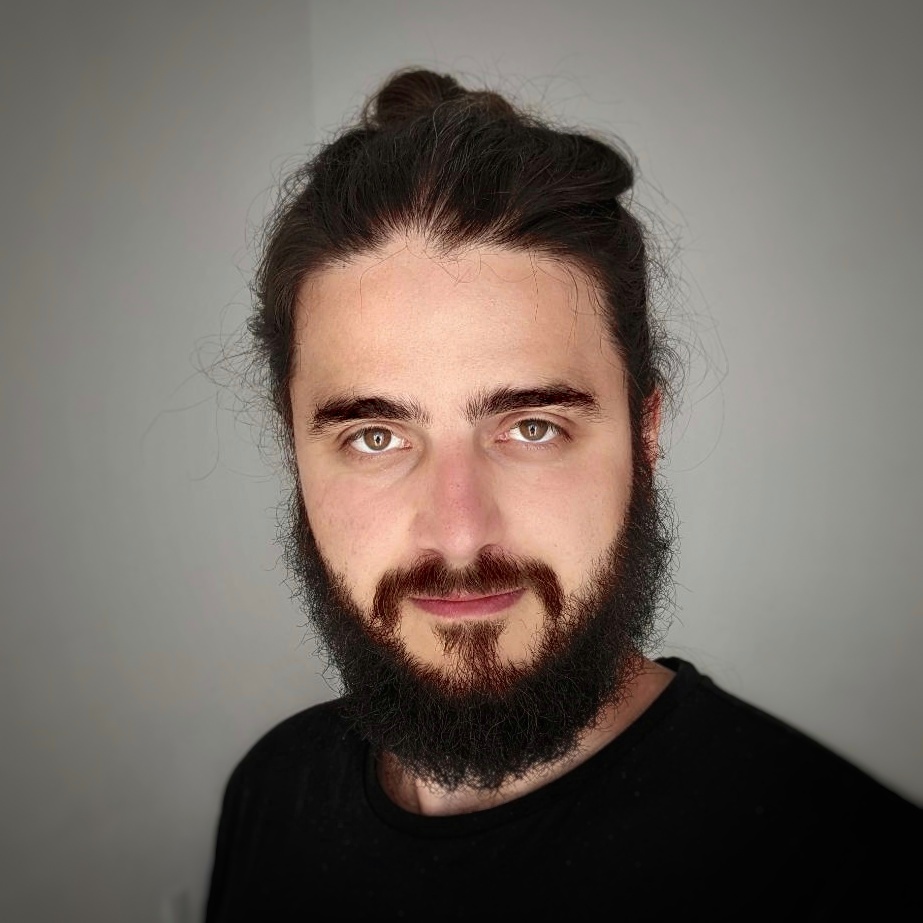
I like to work on:
Topics I'm interested in
Machine learning & AI solutions from prototype to production
I support you and your team with developing robust, high-impact AI and machine learning solutions,
taking them from concept to full-scale production. With expertise across a diverse tech stack, I
ensure the systems we build are not only functional but scalable and reliable in real-world
scenarios.
Tech Stack: Scikit-learn, Keras, PyTorch, TensorFlow, FastAPI, Jupyter, Pandas,
LangChain
MLOps & LLMOps: Operationalizing AI at Scale
I specialize in helping teams implement best practices for managing the machine learning lifecycle.
By setting up comprehensive MLOps pipelines, I ensure your machine learning systems are reliable,
scalable, and easy to maintain. This includes overseeing processes for version control, deployment,
monitoring, and continuous improvement of AI models in production.
Tech Stack: Docker, MLFlow, AWS, Azure, Git, LangSmith
Natural language processing (NLP)
I develop tailored NLP and Generative AI solutions to transform text data into actionable insights
and innovative tools. From tasks like Named Entity Recognition (NER), document classification, and
sentiment analysis to advanced use cases like text generation, conversational AI and (semantic)
search, I help you fully leverage language data for your business.
Tech Stack: HuggingFace Transformers, Sentence-Transformers, LangChain, LLMs,
Scikit-learn, SpaCy, OpenAI, pgvector, LanceDB, Regex, Ollama
Software & Data Engineering
I design and implement software and data solutions that are both maintainable and scalable. From
building data pipelines to developing backend systems, I ensure your infrastructure is optimized for
performance and flexibility, tailored to meet evolving business needs.
Tech Stack: PostgreSQL, SQLite, Redshift, Athena/Presto, SQLAlchemy, Redis,
Elasticsearch, Docker, AWS, Azure, Pytest, FastAPI, Linux, Portainer
You need help with something? Drop me a mail.
Projects
Some open source and commercial projects I work(ed) on.
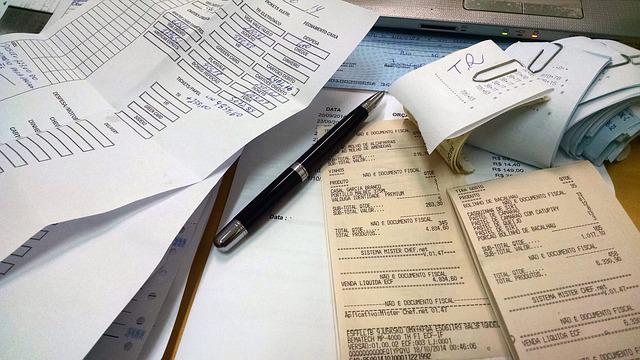
Bookkeeping automation
I worked on building a bookkeeping automation system based on machine learning to handle large numbers of transactions per month.
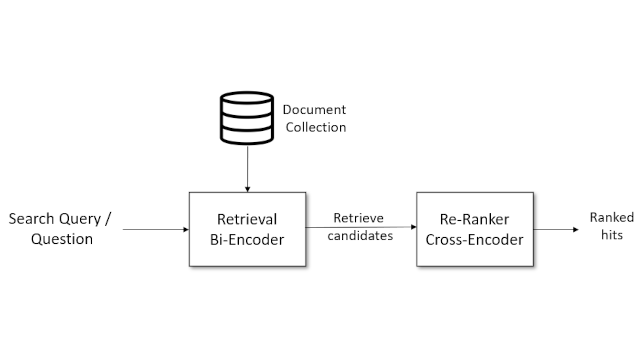
Domain-dependent information retrieval
I worked on a client project to search through a database of domain-specific documents and find semantically close matches.
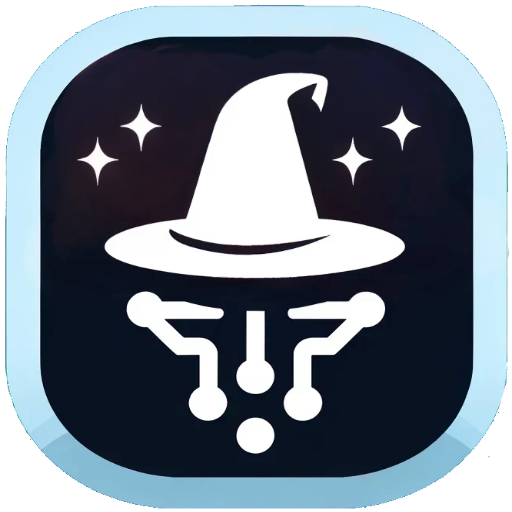
PromptMage
PromptMage is an open source tool that simplifies the process of creating and managing LLM workflows as a self-hosted solution.
Biaslyze
Biaslyze helps to get started with the analysis of bias within NLP models and offers a concrete entry point for further impact assessments and mitigation measures.
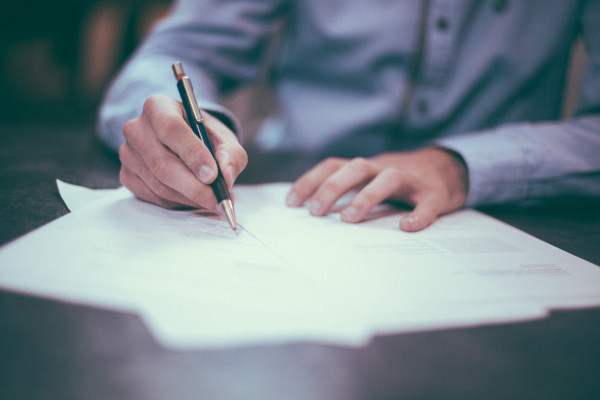
Legal Review of Rental Contracts
Together with dida, I used different methods from the field of NLP to create software that spots errors in rental contracts.
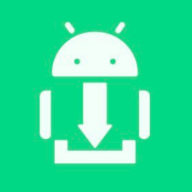
OpenAndroidInstaller
The project helps to keep smartphone up to date with free software. With a graphical installation software, users are easily guided through the installation process of free Android operating systems.
Public speaking
Occasionally, I'm talking about things I work on or give workshops on different
topics.
Some recordings of public talks, podcast appearences and tutorials can be found here.
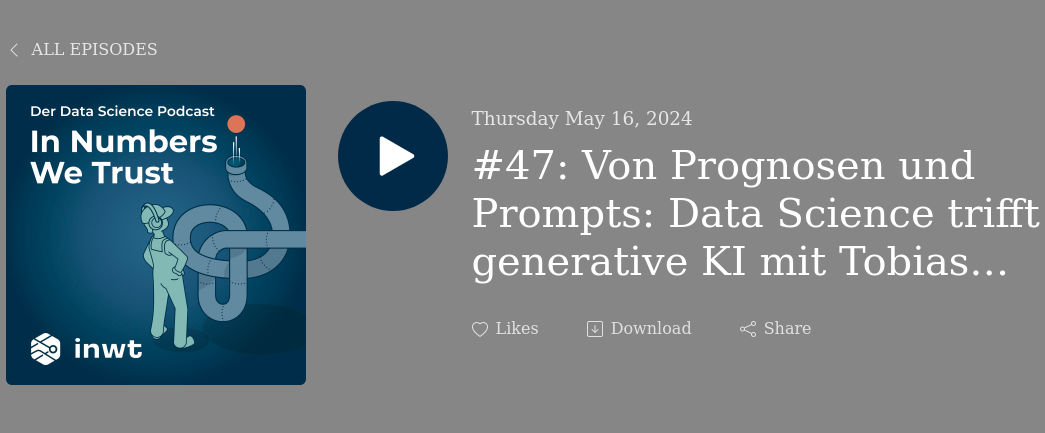
#47 Von Prognosen und Prompts Data Science trifft generative KI mit Tobias Sterbak
In Numbers We Trust - Der Data Science Podcast
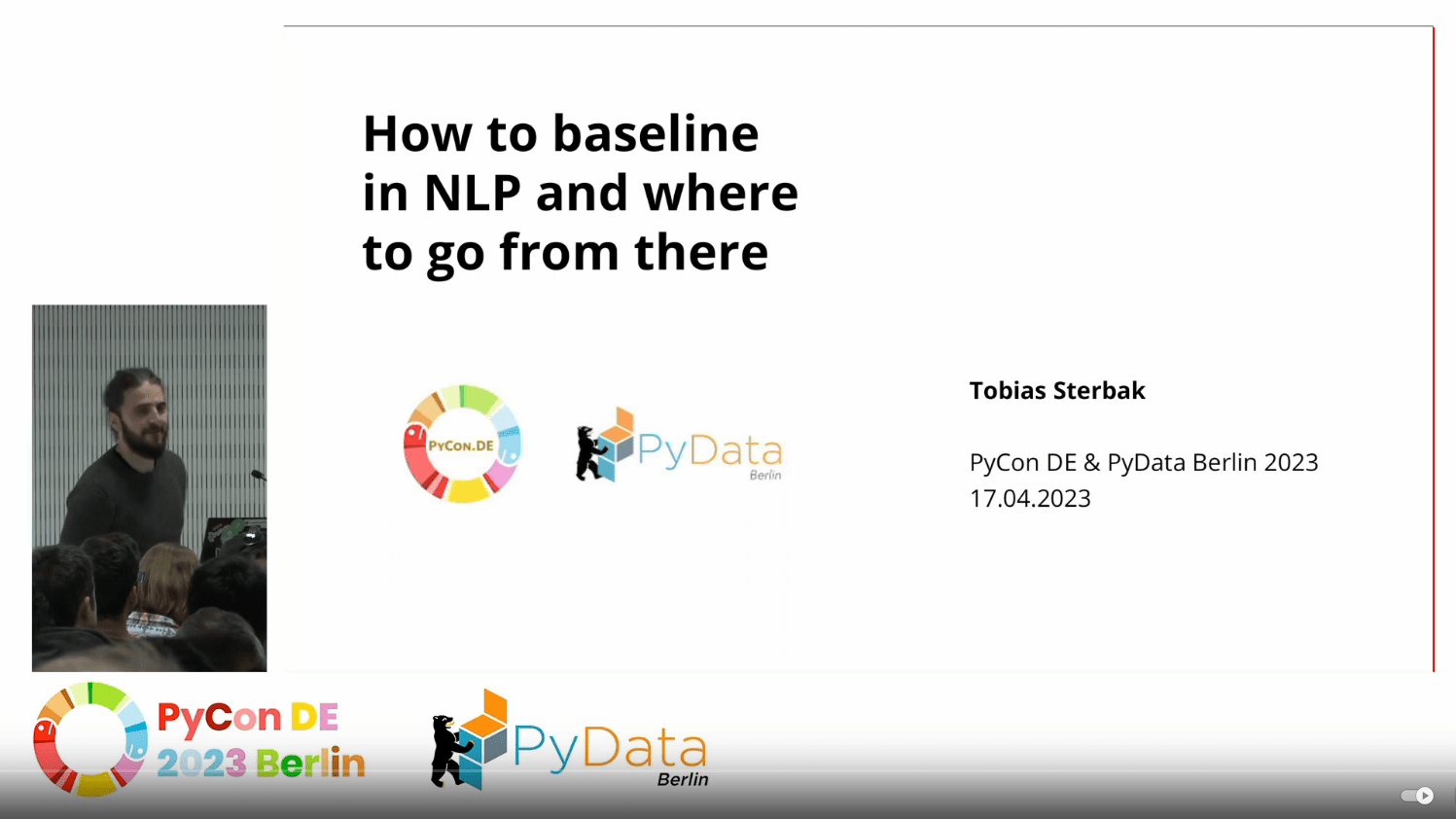
PyConDE & PyData Berlin 2023: How to baseline in NLP and where to go from there
PyCon DE & PyData Berlin 2023
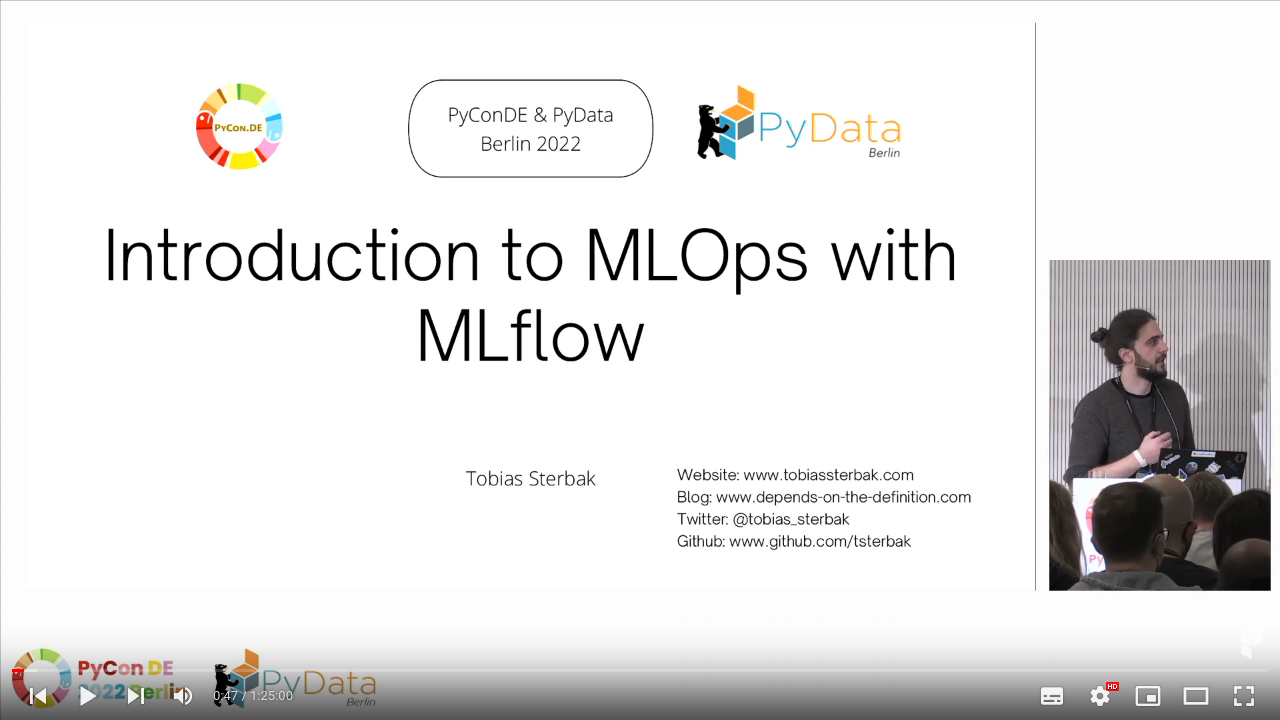
PyConDE & PyData Berlin 2022: Introduction to MLOps with MLflow
PyCon DE & PyData Berlin 2022
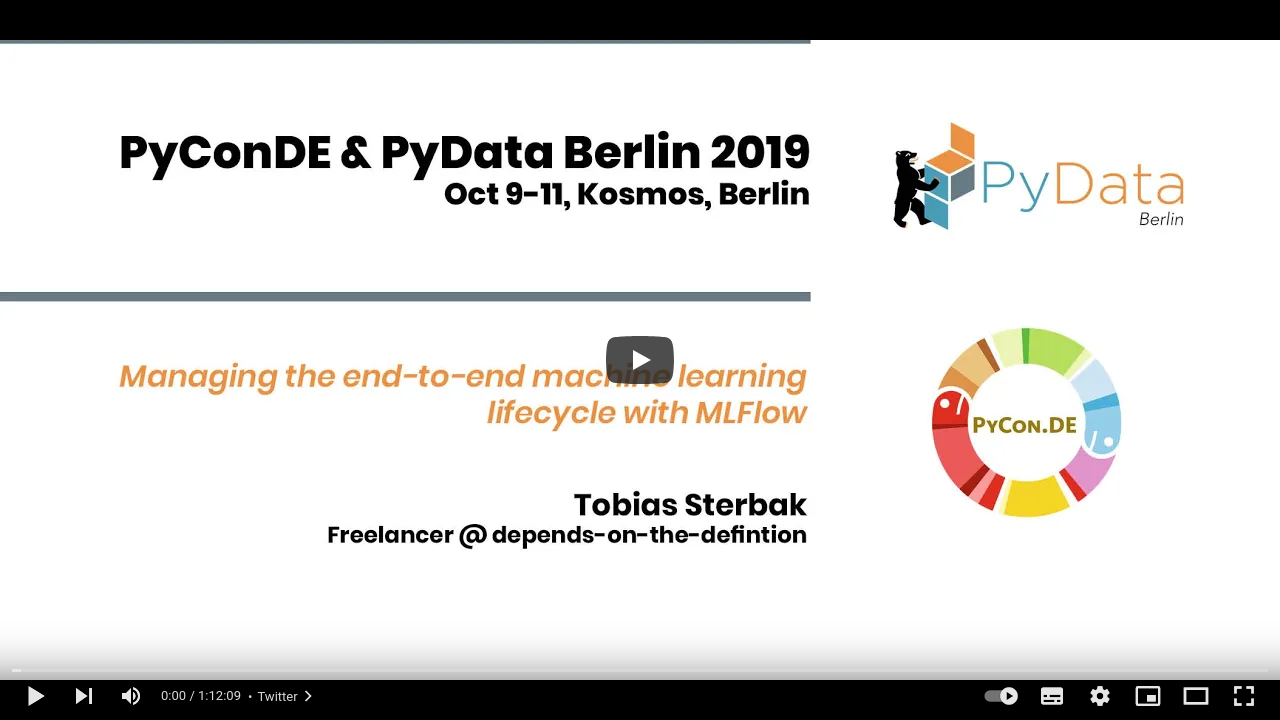
Tutorial: Managing the end-to-end machine learning lifecycle with MLFlow
PyCon DE & PyData Berlin 2019
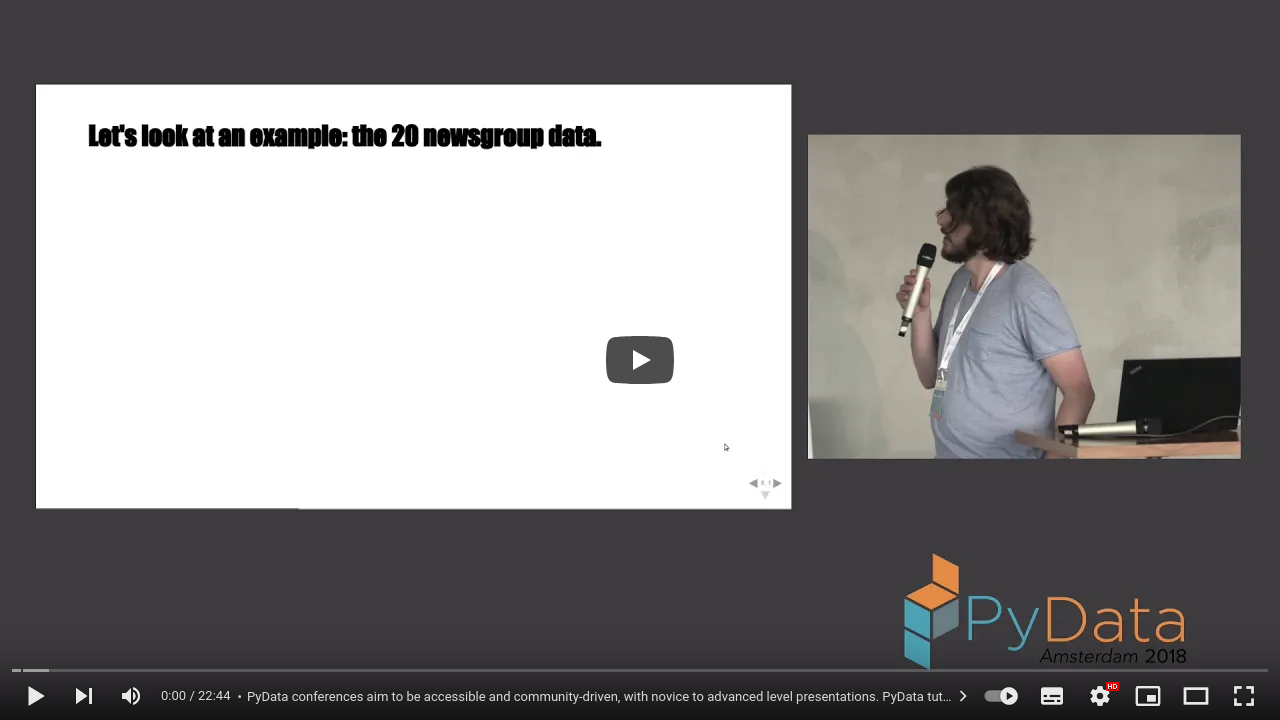
“Why Should I Trust You?” - Debugging black-box text classifiers
PyData Amsterdam 2018
Blog
Selected articles from depends-on-the-definition.com.
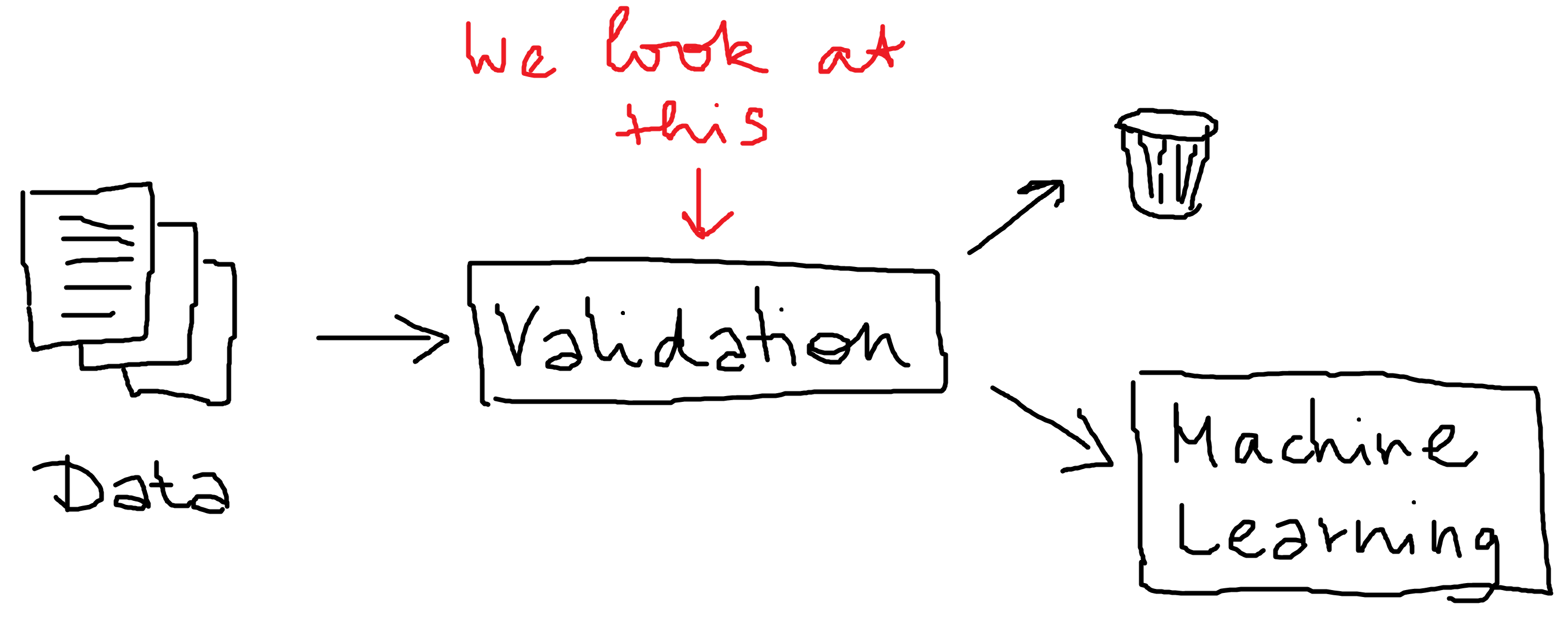
Data validation for NLP applications with topic models
In a recent article, we saw how to implement a basic validation pipeline for text data. Once a machine learning model has been deployed its behavior must be monitored. The predictive performance is expected to degrade over time as the environment changes.
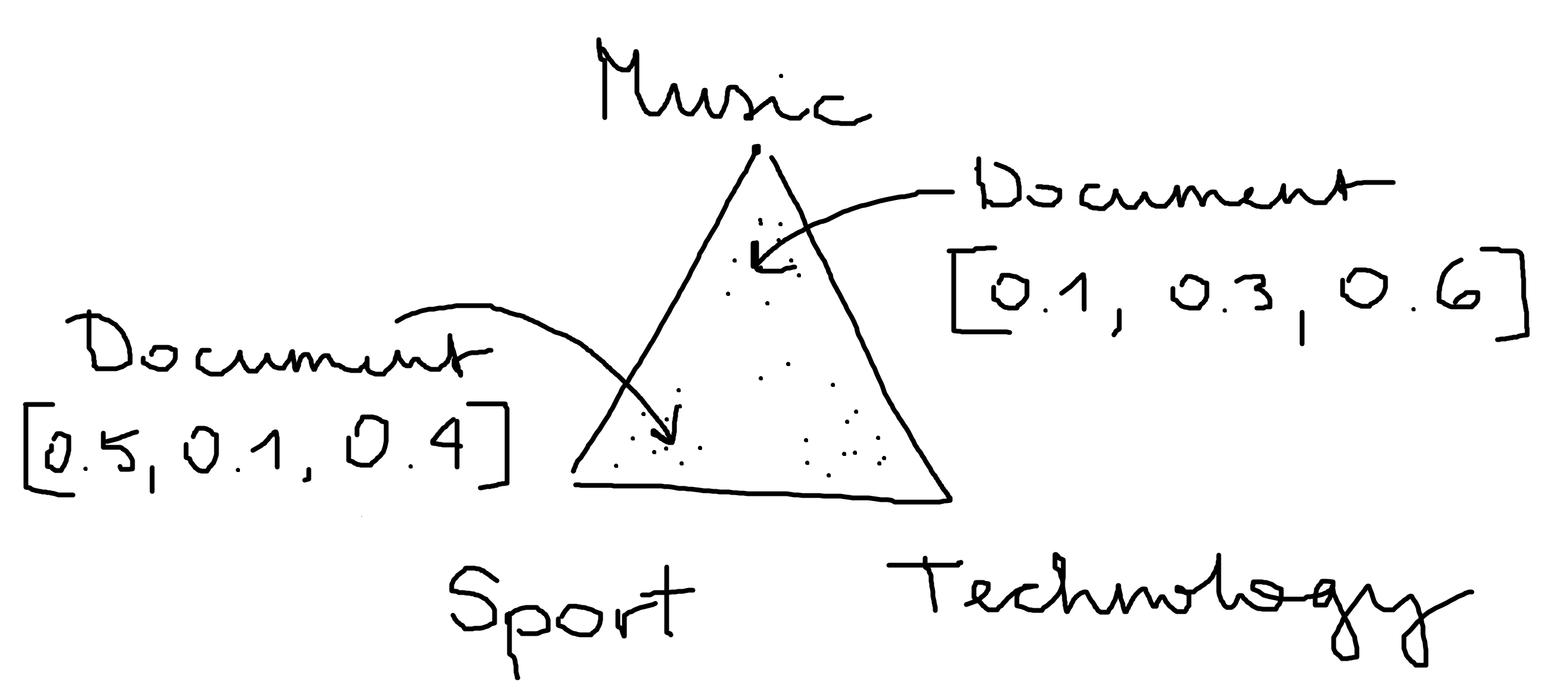
Latent Dirichlet allocation from scratch
Today, I’m going to talk about topic models in NLP. Specifically we will see how the Latent Dirichlet Allocation model works and we will implement it from scratch in numpy.
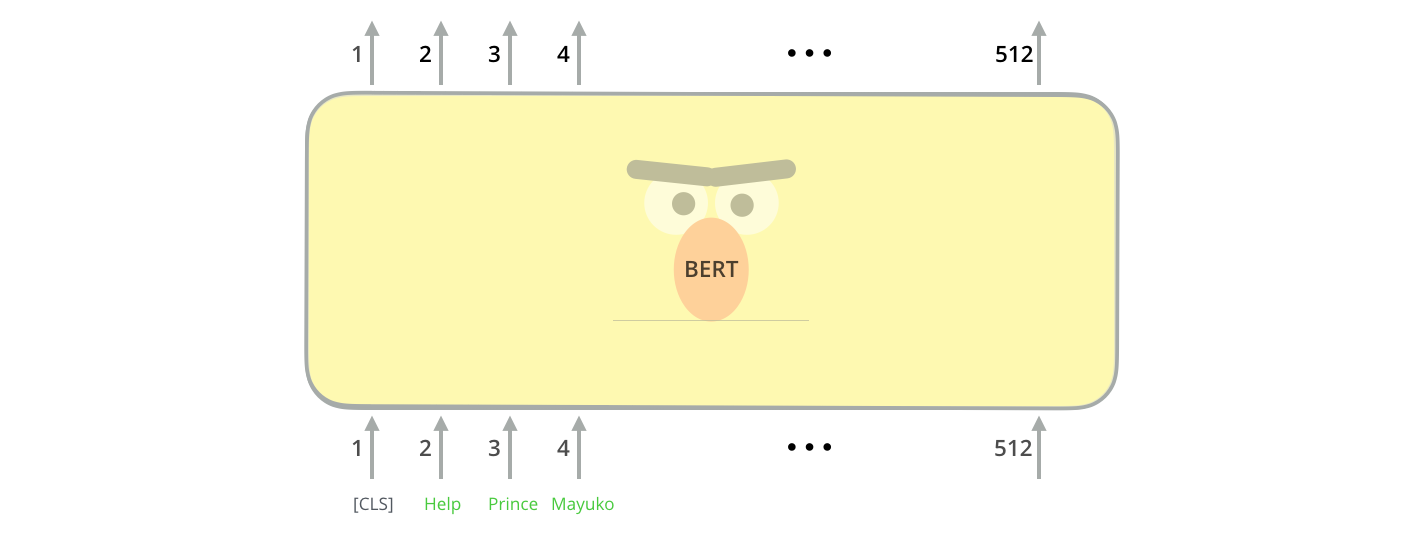
Named entity recognition with Bert
In 2018 we saw the rise of pretraining and finetuning in natural language processing. Large neural networks have been trained on general tasks like language modeling and then fine-tuned for classification tasks. One of the latest milestones in this development is the release of BERT.